
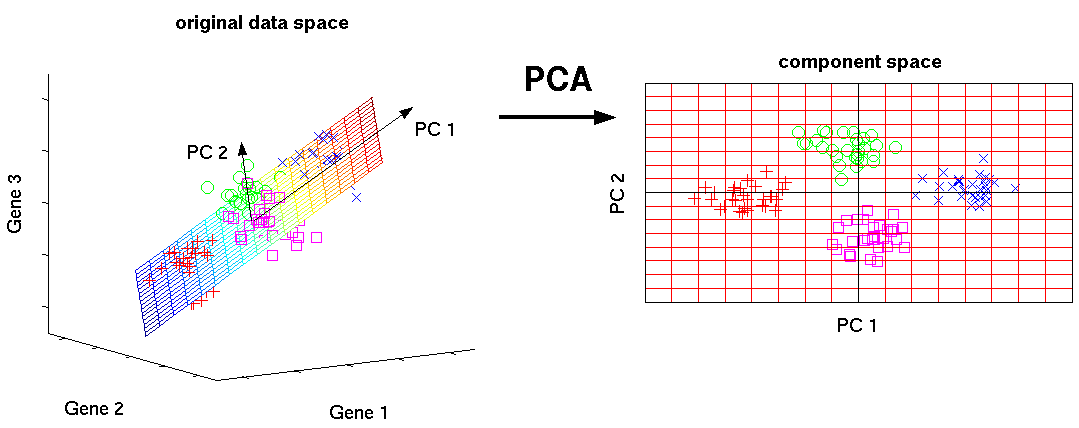
Such knowledge is given by the principal component loadings (graph below). In a PCA model with two components, that is, a plane in K-space, which variables (food provisions) are responsible for the patterns seen among the observations (countries)? We would like to know which variables are influential, and also how the variables are correlated. Colored by geographic location (latitude) of the respective capital city. The first component explains 32% of the variation, and the second component 19%. This provides a map of how the countries relate to each other. The PCA score plot of the first two PCs of a data set about food consumption profiles. A line or plane that is the least squares approximation of a set of data points makes the variance of the coordinates on the line or plane as large as possible. Statistically, PCA finds lines, planes and hyper-planes in the K-dimensional space that approximate the data as well as possible in the least squares sense. The goal is to extract the important information from the data and to express this information as a set of summary indices called principal components. PCA is a very flexible tool and allows analysis of datasets that may contain, for example, multicollinearity, missing values, categorical data, and imprecise measurements. PCA goes back to Cauchy but was first formulated in statistics by Pearson, who described the analysis as finding “ lines and planes of closest fit to systems of points in space”. This overview may uncover the relationships between observations and variables, and among the variables. The most important use of PCA is to represent a multivariate data table as smaller set of variables (summary indices) in order to observe trends, jumps, clusters and outliers. PCA forms the basis of multivariate data analysis based on projection methods. It has been widely used in the areas of pattern recognition and signal processing and is a statistical method under the broad title of factor analysis. Principal component analysis today is one of the most popular multivariate statistical techniques.
PCA METHOD FOR HYPERIMAGE MOVIE
In this project, we try to develop an algorithm which can automatically create aĭataset for abstractive automatic summarization for bigger narrative text bodies suchĪs movie scripts.Using PCA can help identify correlations between data points, such as whether there is a correlation between consumption of foods like frozen fish and crisp bread in Nordic countries. Therefore, creating datasets from larger texts can help improve automatic summarization. The bigger theĭifference in size between the summary and the original text, the harder the problem willīe since important information will be sparser and identifying them can be more difficult. Papers, which are somewhat small texts with simple and clear structure. Most researchĪbout automatic summarization revolves around summarizing news articles or scientific ItĬan be used to create the data needed to train different language models. %X Automatic text alignment is an important problem in natural language processing. %T AligNarr: Aligning Narratives of Different Length for Movie Summarization : International Max Planck Research School, MPI for Informatics, Max Planck Societyĭatabases and Information Systems, MPI for Informatics, Max Planck Society
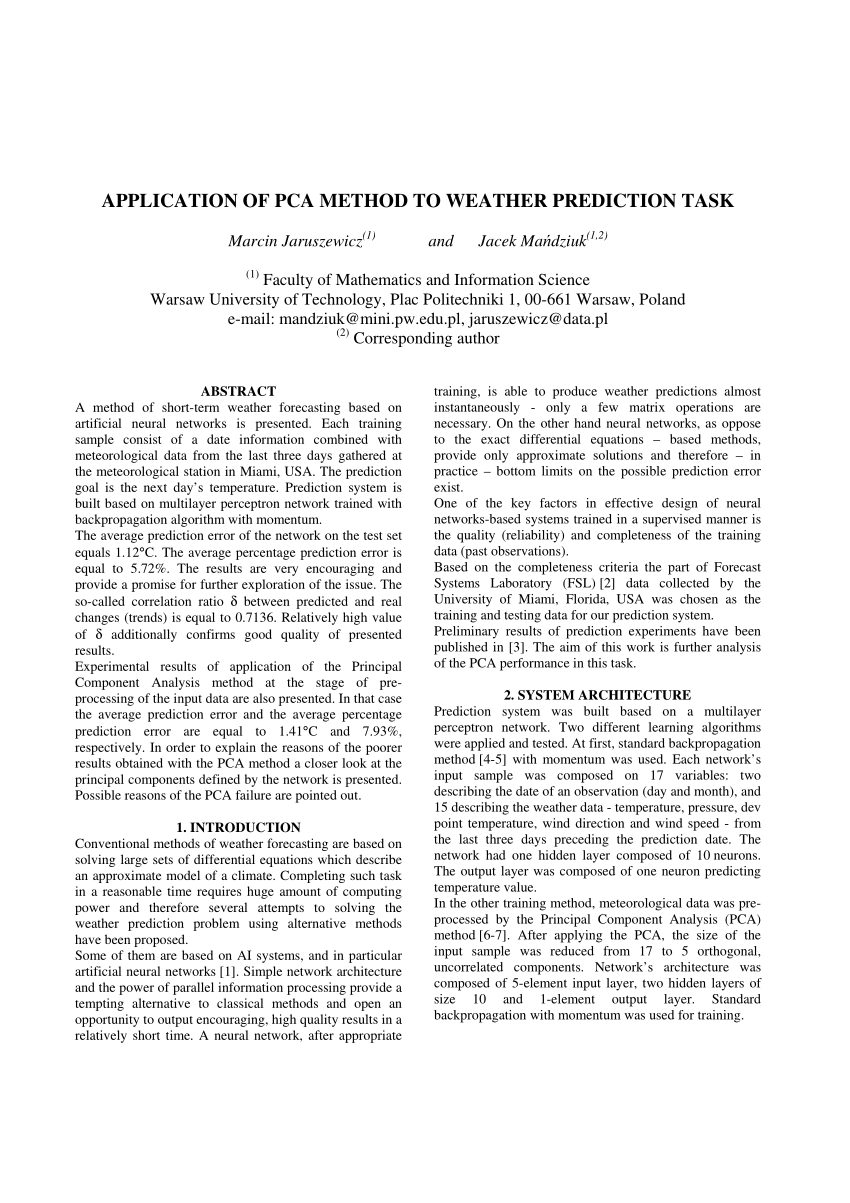
%+ Databases and Information Systems, MPI for Informatics, Max Planck Society Intrinsic evaluation shows the superior size and quality of the Ascent KB, and an extrinsic evaluation for QA-support tasks underlines the benefits of = , Ascent combines open information extraction with judicious cleaning using language models. The latter are important to express temporal and spatial validity of assertions and further qualifiers. Ascent goes beyond triples by capturing composite concepts with subgroups and aspects, and by refining assertions with semantic facets. This thesis presents a methodology, called Ascent, to automatically build a large-scale knowledge base (KB) of CSK assertions, with advanced expressiveness and both better precision and recall than prior works. Also, these projects have either prioritized precision or recall, but hardly reconcile these complementary goals. Prior works like ConceptNet, TupleKB and others compiled large CSK collections, but are restricted in their expressiveness to subject-predicate-object (SPO) triples with simple concepts for S and monolithic strings for P and O. Commonsense knowledge (CSK) about concepts and their properties is useful for AI applications such as robust chatbots.
